Can AI Close the Learning Gap? The Role of Adaptive Learning Technologies
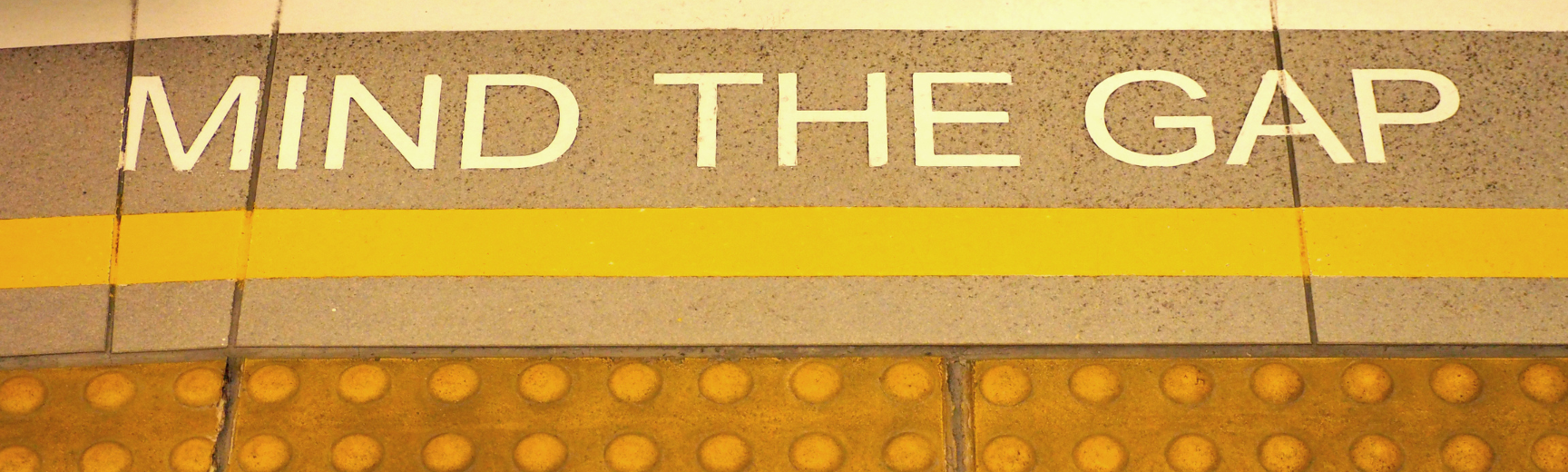
Education has long been heralded as the great equalizer, yet persistent disparities in learning outcomes continue to plague students worldwide. Socioeconomic status, geographic location, and access to quality instruction all contribute to a widening achievement gap (Reardon, 2011). In recent years, Artificial Intelligence (AI) has been touted as a potential solution, particularly through the emergence of adaptive learning technologies (Joshi, 2023), AI-driven systems that personalize instruction based on a student's unique needs.
But can AI truly close the learning gap, or is it just another technological innovation that will reinforce existing inequalities? This article explores the promises, limitations, and ethical dilemmas of using AI to foster educational equity.
The Promise of Adaptive Learning Technologies
Adaptive learning technologies leverage machine learning algorithms, natural language processing, and real-time analytics to tailor educational experiences to individual students. Unlike traditional one-size-fits-all instruction, these systems continuously analyze student performance and dynamically adjust content, difficulty levels, and instructional strategies (Luckin et al., 2016).
One of the primary advantages of AI-driven education is personalization at scale. Traditional classrooms often struggle to meet the diverse needs of students, especially those from underserved backgrounds who may enter school with fewer academic resources. AI-based systems such as Knewton, DreamBox, and Carnegie Learning adapt lessons in real-time, ensuring that students receive targeted instruction at their optimal level of challenge (Pane et al., 2014).
Research suggests that early learning disparities between students from low-income and high-income backgrounds stem from differential access to high-quality instruction and resources (Reardon, 2011). AI-driven platforms provide students with on-demand, personalized tutoring, potentially compensating for the lack of educational support at home. Additionally, AI can automate grading, generate individualized feedback, and recommend resources, alleviating the burden on teachers in underfunded schools (Anjum, 2023).
Adaptive technologies hold particular promise for students with learning disabilities and English language learners. AI-powered speech recognition and text-to-speech tools can provide real-time support, making content more accessible. Furthermore, cognitive tutors such as ASSISTments and ALEKS use error analysis to identify misconceptions and offer targeted interventions (Koedinger et al., 2013).
Challenges and Ethical Concerns
Despite its potential, the integration of AI in education is not without its challenges. Issues of data privacy, algorithmic bias, and accessibility raise critical questions about whether adaptive learning technologies can truly be a force for equity.
AI systems are only as unbiased as the data they are trained on. If historical data reflects existing educational disparities, adaptive learning technologies may reinforce rather than eliminate biases. For instance, studies have shown that AI-driven assessments can underpredict the capabilities of marginalized students if not carefully calibrated (O’Neil, 2016).
While AI-powered education platforms offer immense potential, they require reliable internet access and digital literacy. In many low-income communities and developing regions, students lack access to the very tools necessary to benefit from AI-driven learning (Selwyn, 2020). Without addressing these infrastructural challenges, AI may widen rather than bridge the learning gap.
AI therefore should not be seen as a replacement for teachers but as an augmentative tool that enhances instructional practices. Research emphasizes that the most effective educational interventions blend human expertise with AI-driven insights (Holmes et al., 2019). Teachers play a crucial role in interpreting AI recommendations, addressing students' emotional and social needs, and ensuring that technology does not replace meaningful human interaction in learning.
The Path Forward: Ethical and Inclusive AI in Education
For AI to truly close the learning gap, policymakers, educators, and technologists must prioritize equity, transparency, and inclusivity in the design and implementation of adaptive learning technologies.
- Bias Mitigation in AI Models: Developers must ensure that training datasets are diverse and representative, with rigorous testing for biases in AI predictions.
- Infrastructure Investment: Governments and institutions must bridge the digital divide by investing in high-speed internet, affordable devices, and digital literacy programs.
- Human-AI Synergy: AI should be integrated alongside teachers, not in place of them, ensuring a human-centered approach to adaptive learning.
- Data Privacy Protections: Policies must be enacted to protect student data from misuse, ensuring transparency in how AI-driven platforms collect and utilize information.
Conclusion: The Potential for Transformation
Adaptive learning technologies hold immense potential to transform education, making it more personalized, accessible, and effective especially for under-resourced communities. However, the idea that AI alone can close the learning gap is overly simplistic and seemingly unrealistic. Without addressing systemic barriers, such as poverty, resource allocation, and teacher training, AI will remain a tool that benefits the privileged rather than an equalizing force. The real question is not whether AI can close the learning gap, but whether we as educators, policymakers, and society will ensure that it is implemented ethically, equitably, and inclusively. If done right, AI can be a powerful ally in the quest for educational justice.
References
Anjum, P.G., Choubey, P.J., Kushwaha, S., & Patkar, V. (2023). AI in Education: Evaluating the Efficacy and Fairness of Automated Grading Systems. International Journal of Innovative Research in Science, Engineering and Technology, 12(6), 9040-9050.
Holmes, W., Bialik, M., & Fadel, C. (2019). Artificial Intelligence in Education: Promises and Implications for Teaching and Learning. Center for Curriculum Redesign.
Koedinger, K. R., Corbett, A. T., & Perfetti, C. (2013). The Knowledge-Learning-Instruction Framework: Bridging the Science-Practice Chasm to Enhance Robust Student Learning. Cognitive Science, 37(5), 757–798.
Luckin, R., Holmes, W., Griffiths, M., & Forcier, L. B. (2016). Intelligence Unleashed: An Argument for AI in Education. Pearson.
Joshi, M. A. (2024). Adaptive learning through artificial intelligence. Joshi, MA, 41-43.
O’Neil, C. (2016). Weapons of Math Destruction: How Big Data Increases Inequality and Threatens Democracy. Crown.
Pane, J. F., Griffin, B. A., McCaffrey, D. F., & Karam, R. (2014). Effectiveness of Cognitive Tutor Algebra I at Scale. Educational Evaluation and Policy Analysis, 36(2), 127–144.
Reardon, S. F. (2011). The Widening Academic Achievement Gap Between the Rich and the Poor: New Evidence and Possible Explanations. Russell Sage Foundation Journal of the Social Sciences, 2(2), 34–57.
Selwyn, N. (2019). Should robots replace teachers?: AI and the future of education. John Wiley & Sons.
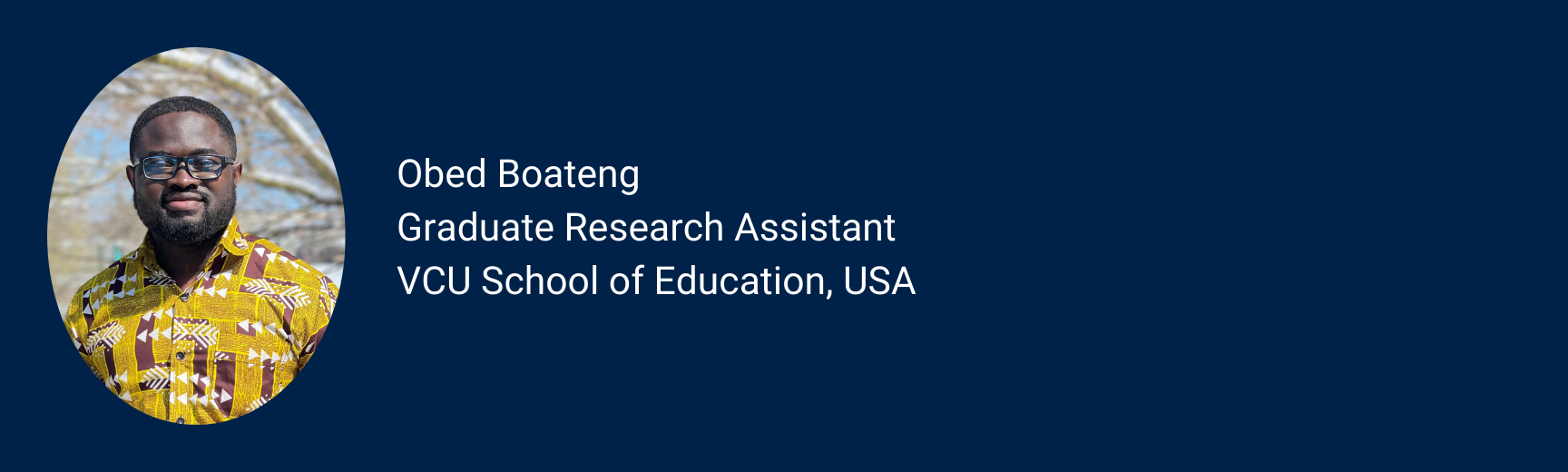
The content expressed in this blog is that of the author(s) and does not necessarily reflect the position of the website owner. All content provided is shared in the spirit of knowledge exchange with our AIEOU community of practice. The author(s) retains full ownership of the content, and the website owner is not responsible for any errors or omissions, nor for the ongoing availability of this information. If you wish to share or use any content you have read here, please ensure to cite the author appropriately. Thank you for respecting the author's intellectual property.
Suggested citation: Boateng, O. (2025, 4 March). Can AI Close the Learning Gap? The Role of Adaptive Learning Technologies. AIEOU.